

The most popular method to find overlapping communities is the Clique Percolation Method described by Palla et al. the Potts model) or synchronization models. Different kind of approaches have also been developed, including ones utilizing spectral functions of the graphs, , dynamic algorithms like random walks, , spin models (e.g. In a short time the Q function evaluating the goodness of partitioning a graph into given clusters became an essential element of a wide range of clustering methods. Later they introduced the modularity measure, Q with which the optimal number of removed edges could be determined. In 2002 Girvan and Newman published a seminal paper using an algorithm for detecting communities by iteratively removing edges of high betweenness centrality values from the network, and defining communities as the connected components of the network after these edge removals. At the moment there is no ‘best method’ available to find network modules, and even the widely used algorithms may suffer from serious problems (see Figure S1.1, and Tables S1.1 and S1.2 in the Electronic Supplementary Material S1) –, although they usually provide useful and clear dissections of networks. So far a wide variety of great ideas and powerful approaches based on very different physical or algorithmic grounds were applied in order to solve this problem. The development of a method, which translates this intuitive definition of modules into a practically applicable, fast, accurate and widely usable algorithm turned out to be a very challenging problem. Numerous module determination methods are based on the intuitive picture identifying the network communities as dense groups of the network, whose nodes have a much stronger influence on each other than on the rest of the network. In real networks, module or community structure plays a central role in the understanding of topology and dynamics. The funders had no role in study design, data collection and analysis, decision to publish, or preparation of the manuscript.Ĭompeting interests: The authors have declared that no competing interests exist. This is an open-access article distributed under the terms of the Creative Commons Attribution License, which permits unrestricted use, distribution, and reproduction in any medium, provided the original author and source are credited.įunding: This research was supported by research grants from the EU (FP6-518230), Hungarian Science Foundation (OTKA K-69105) and by an unrestricted grant from Unilever Hungary to the Hungarian Student Research Foundation, which helped the research of the authors. Received: MaAccepted: AugPublished: September 2, 2010Ĭopyright: © 2010 Kovács et al. PLoS ONE 5(9):Įditor: Olaf Sporns, Indiana University, United States of America degree or clustering index).Citation: Kovács IA, Palotai R, Szalay MS, Csermely P (2010) Community Landscapes: An Integrative Approach to Determine Overlapping Network Module Hierarchy, Identify Key Nodes and Predict Network Dynamics. Finally, to enrich the visual exploration, it is possible to visually render local topological properties of the network (e.g. The plugin also allows the extraction of parts of the network that contain a selected subset of reactions. the organism or perturbation under study), we propose facilities to edit/select putative biochemical transformations. Since the definition of this list is closely related to experimentation (i.e. Inference requires a list of potential biochemical transformations. Here we present a new plugin for Cytoscape dedicated to the inference and visualization of high-resolution metabolomic networks.
Cytoscape 2.8 software#
There is currently no available software that allows inference and visualization of such high-resolution metabolomic networks directly from raw data. To analyze, explore and interpret these two kinds of relations, powerful visualization tools are required.

The combination of these two inference methods generates networks containing hundreds of nodes (metabolites) and hundreds of predicted edges (biochemical reactions and/or high correlations). Moreover, perturbation studies allow the use of correlation analysis to infer/confirm links between metabolites that correlate across various conditions.
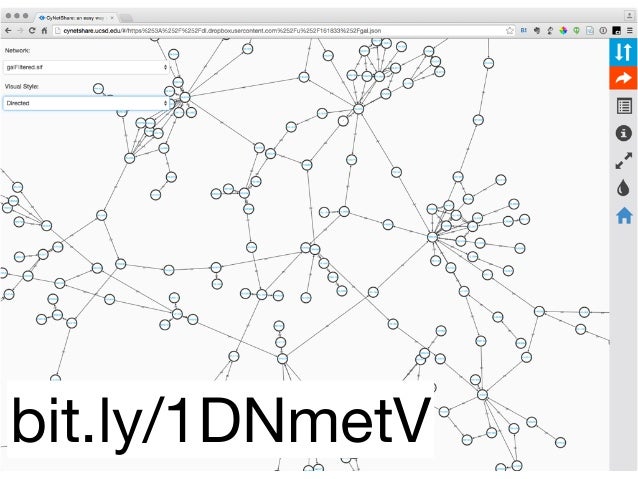
Such high-resolution data has also been used to predict ab initio biochemical interactions between metabolites. Recently, ultra high-resolution mass spectrometry (FTICR-MS or Orbitrap) has been successfully used in metabolomic studies. Various spectrometric technologies are capable of identifying thousands of metabolites. Metabolomics aims at the identification and quantification of all metabolites that are present in a cell, tissue or biofluid at a given moment and under particular conditions.
